Is Scaling the Key to Achieving Artificial General Intelligence (AGI)?
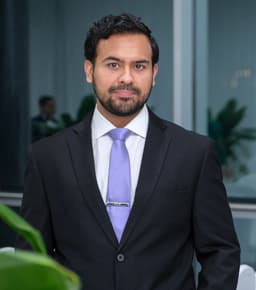
Javier Reyes
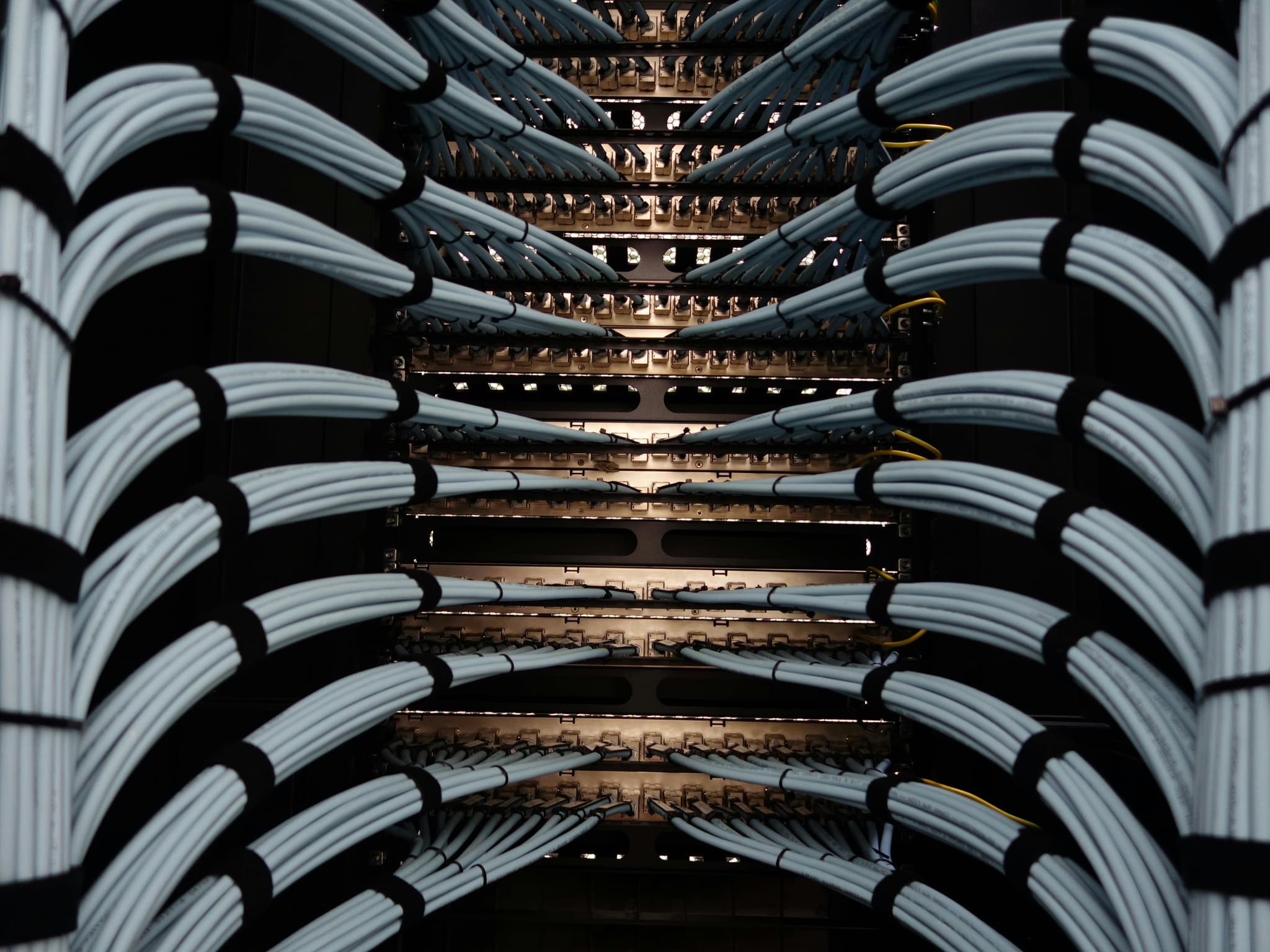
Achieving Artificial General Intelligence (AGI) represents the ultimate goal of AI research, promising a transformative leap in technology. Unlike narrow AI systems that excel at specific tasks, AGI aspires to replicate the broad intellectual abilities of humans, revolutionizing industries and redefining problem-solving capabilities. This pursuit has ignited substantial debate, with scaling—increasing model size, computational power, and data—emerging as a potentially pivotal strategy in unlocking AGI.
The Argument for Scaling
In recent years, breakthroughs in large-scale models like OpenAI’s GPT series and DeepMind’s Gato have fueled the belief that scaling plays a pivotal role in advancing AI capabilities. These models demonstrate that increasing the number of parameters and training on massive datasets can significantly enhance performance across a wide range of tasks. Proponents of this approach argue that scaling:
Improves Generalization: Larger models trained on diverse datasets exhibit emergent capabilities, solving tasks that weren’t explicitly programmed.
Reduces the Need for Human Intervention: By training on vast and varied data, scaled models learn to perform tasks without requiring task-specific fine-tuning.
Aligns with Biological Analogies: The human brain, often considered the benchmark for AGI, contains approximately 86 billion neurons. Scaling AI models could mimic the complexity of neural connections and information processing.
The Role of Infrastructure Investments
Scaling AI to AGI demands immense computational resources, driving major investments in state-of-the-art data centers. Microsoft, which hosts most of OpenAI's digital infrastructure, spent over $50 billion last year on capital expenditures, primarily for data center construction, and has committed an additional $80 billion for this fiscal year to further develop AI-enabled data centers(source). These facilities provide the critical infrastructure needed for training and deploying the massive models essential for AGI research.
On the other hand, Meta's capital expenditures for the full year 2024 are projected to range between $38 billion and $40 billion(source). As part of this substantial investment, Meta has been rethinking its data center layouts to better support its AI ambitions. One example is the Temple Campus in Texas, which is designed specifically for AI workloads and expected to be completed in Q1 2025. This facility features a cutting-edge layout with high-density compute infrastructure and advanced cooling systems, optimized to handle the significant power demands of large-scale AI operations efficiently.
To ensure these operations have a sustainable and reliable energy supply, Meta has already secured 1,919 MW of power purchase agreements (PPAs) in Texas. These agreements, sourced from providers such as EDP Renewables, ENGIE, Pine Gate, Ares Management, Citigroup Energy, and Longroad Energy(source), will deliver clean energy to power both the Temple Campus and other facilities. Through these strategic investments in innovative infrastructure and renewable energy, Meta is positioning itself to lead in AI while maintaining a strong commitment to sustainability.
Challenges in Solely Relying on Scaling
Despite its promise, scaling alone has limitations and significant challenges:
Diminishing Returns: Beyond a certain point, merely increasing model size may yield marginal improvements relative to the computational and financial costs involved.
Energy Consumption: Training massive models requires enormous energy resources, raising concerns about sustainability and environmental impact.
Lack of True Understanding: Current large-scale models, despite their impressive outputs, often fail to exhibit true understanding or reasoning, essential components of AGI.
Infrastructure Risks: For companies relying on partnerships rather than direct ownership, such as OpenAI with Microsoft and Oracle, there may still be challenges in optimizing long-term scalability and managing costs effectively.
Conclusion
While scaling has played a pivotal role in advancing AI capabilities, achieving AGI will require a more holistic approach. The massive investments from companies like Microsoft and Meta in data center infrastructure and renewable energy are critical steps, but true progress lies in combining computational scale with innovations in algorithmic design, hardware optimization, high-quality data, and interdisciplinary research. These advancements must also address challenges such as sustainability and system interpretability. For businesses investing in AI-driven infrastructure, the path to AGI represents both immense potential and significant hurdles. By leveraging Aterio’s comprehensive data sets, companies can better understand the evolving landscape, identify key opportunities, and stay ahead in the race toward the future of AI.